Modelling hip hop songs dataset using PCA
An example of PCA based modelling approach
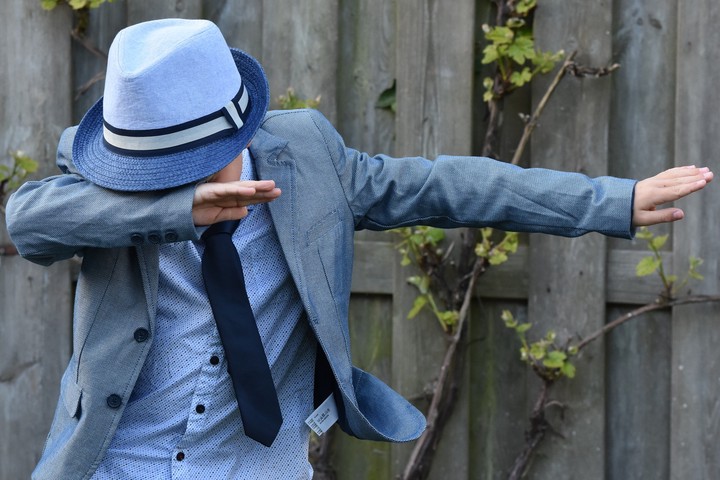
This dataset was publisged in tidy tuesday a few days back, The data contains details of hiphop songs and their ratings given by few independent critics. Some other details about the critics are also given such as gender. Details such as year, title, artist has been given about the songs.
Lets load the data
rankings <- read_csv("https://raw.githubusercontent.com/rfordatascience/tidytuesday/master/data/2020/2020-04-14/rankings.csv")
## Parsed with column specification:
## cols(
## ID = col_double(),
## title = col_character(),
## artist = col_character(),
## year = col_double(),
## gender = col_character(),
## points = col_double(),
## n = col_double(),
## n1 = col_double(),
## n2 = col_double(),
## n3 = col_double(),
## n4 = col_double(),
## n5 = col_double()
## )
Explore the data
rankings %>%
ggplot(aes(year, points, color = gender)) +
geom_jitter(alpha = 0.7) +
scale_y_log10()
rankings %>%
count(gender, wt = points, sort = T)
## # A tibble: 3 x 2
## gender n
## <chr> <dbl>
## 1 male 2870
## 2 female 192
## 3 mixed 148
Setting up spotify developer account to access API.
#library(spotifyr)
#Sys.setenv(SPOTIFY_CLIENT_ID = 'XXXXXXXXXXXXXXXXXXXXXXXXXXXXXXXXX')
#Sys.setenv(SPOTIFY_CLIENT_SECRET = 'XXXXXXXXXXXXXXXXXXXXXXXXXXXXXX')
access_token <- spotifyr::get_spotify_access_token()
Fetching hiphop songs playlist with features
playlist_features <-spotifyr::get_playlist_audio_features("tmock1923", "7esD007S7kzeSwVtcH9GFe")
glimpse(playlist_features)
## Rows: 250
## Columns: 61
## $ playlist_id <chr> "7esD007S7kzeSwVtcH9GFe", "7esD0...
## $ playlist_name <chr> "Top 250 Hiphop", "Top 250 Hipho...
## $ playlist_img <chr> "https://mosaic.scdn.co/640/ab67...
## $ playlist_owner_name <chr> "tmock1923", "tmock1923", "tmock...
## $ playlist_owner_id <chr> "tmock1923", "tmock1923", "tmock...
## $ danceability <dbl> 0.873, 0.797, 0.763, 0.947, 0.77...
## $ energy <dbl> 0.816, 0.582, 0.786, 0.607, 0.46...
## $ key <int> 9, 2, 10, 10, 10, 11, 1, 4, 6, 6...
## $ loudness <dbl> -4.645, -12.970, -6.472, -10.580...
## $ mode <int> 1, 1, 0, 0, 0, 0, 1, 0, 0, 1, 0,...
## $ speechiness <dbl> 0.2560, 0.2550, 0.2290, 0.2020, ...
## $ acousticness <dbl> 0.483000, 0.004840, 0.014600, 0....
## $ instrumentalness <dbl> 0.00e+00, 2.04e-06, 1.14e-02, 4....
## $ liveness <dbl> 0.1460, 0.5170, 0.0817, 0.0861, ...
## $ valence <dbl> 0.790, 0.415, 0.504, 0.732, 0.79...
## $ tempo <dbl> 96.067, 105.974, 93.857, 100.619...
## $ track.id <chr> "2b7FqlHc3JrzlYtGEkzq22", "1yo16...
## $ analysis_url <chr> "https://api.spotify.com/v1/audi...
## $ time_signature <int> 4, 4, 4, 4, 4, 4, 4, 4, 4, 4, 4,...
## $ added_at <chr> "2020-04-14T16:16:07Z", "2020-04...
## $ is_local <lgl> FALSE, FALSE, FALSE, FALSE, FALS...
## $ primary_color <lgl> NA, NA, NA, NA, NA, NA, NA, NA, ...
## $ added_by.href <chr> "https://api.spotify.com/v1/user...
## $ added_by.id <chr> "tmock1923", "tmock1923", "tmock...
## $ added_by.type <chr> "user", "user", "user", "user", ...
## $ added_by.uri <chr> "spotify:user:tmock1923", "spoti...
## $ added_by.external_urls.spotify <chr> "https://open.spotify.com/user/t...
## $ track.artists <list> [<data.frame[1 x 6]>, <data.fra...
## $ track.available_markets <list> [<"AD", "AE", "AR", "AT", "AU",...
## $ track.disc_number <int> 1, 1, 1, 1, 1, 1, 1, 1, 1, 1, 1,...
## $ track.duration_ms <int> 301466, 282640, 325506, 431800, ...
## $ track.episode <lgl> FALSE, FALSE, FALSE, FALSE, FALS...
## $ track.explicit <lgl> TRUE, FALSE, TRUE, FALSE, FALSE,...
## $ track.href <chr> "https://api.spotify.com/v1/trac...
## $ track.is_local <lgl> FALSE, FALSE, FALSE, FALSE, FALS...
## $ track.name <chr> "Juicy - 2007 Remaster", "Fight ...
## $ track.popularity <int> 62, 65, 74, 50, 18, 73, 68, 67, ...
## $ track.preview_url <chr> "https://p.scdn.co/mp3-preview/f...
## $ track.track <lgl> TRUE, TRUE, TRUE, TRUE, TRUE, TR...
## $ track.track_number <int> 1, 20, 15, 7, 1, 8, 8, 12, 23, 9...
## $ track.type <chr> "track", "track", "track", "trac...
## $ track.uri <chr> "spotify:track:2b7FqlHc3JrzlYtGE...
## $ track.album.album_type <chr> "compilation", "album", "album",...
## $ track.album.artists <list> [<data.frame[1 x 6]>, <data.fra...
## $ track.album.available_markets <list> [<"AD", "AE", "AR", "AT", "AU",...
## $ track.album.href <chr> "https://api.spotify.com/v1/albu...
## $ track.album.id <chr> "5XqEf16OrHdmMoNS1b6WDg", "0aFNb...
## $ track.album.images <list> [<data.frame[3 x 3]>, <data.fra...
## $ track.album.name <chr> "Greatest Hits", "Fear Of A Blac...
## $ track.album.release_date <chr> "2007-03-05", "1990-04-10", "199...
## $ track.album.release_date_precision <chr> "day", "day", "day", "day", "day...
## $ track.album.total_tracks <int> 17, 20, 16, 8, 10, 15, 14, 16, 4...
## $ track.album.type <chr> "album", "album", "album", "albu...
## $ track.album.uri <chr> "spotify:album:5XqEf16OrHdmMoNS1...
## $ track.album.external_urls.spotify <chr> "https://open.spotify.com/album/...
## $ track.external_ids.isrc <chr> "USBB40706421", "USDJ29000034", ...
## $ track.external_urls.spotify <chr> "https://open.spotify.com/track/...
## $ video_thumbnail.url <lgl> NA, NA, NA, NA, NA, NA, NA, NA, ...
## $ key_name <chr> "A", "D", "A#", "A#", "A#", "B",...
## $ mode_name <chr> "major", "major", "minor", "mino...
## $ key_mode <chr> "A major", "D major", "A# minor"...
glimpse(rankings)
## Rows: 311
## Columns: 12
## $ ID <dbl> 1, 2, 3, 4, 5, 6, 7, 8, 9, 10, 11, 12, 13, 14, 15, 16, 17, 1...
## $ title <chr> "Juicy", "Fight The Power", "Shook Ones (Part II)", "The Mes...
## $ artist <chr> "The Notorious B.I.G.", "Public Enemy", "Mobb Deep", "Grandm...
## $ year <dbl> 1994, 1989, 1995, 1982, 1992, 1993, 1993, 1992, 1994, 1995, ...
## $ gender <chr> "male", "male", "male", "male", "male", "male", "male", "mal...
## $ points <dbl> 140, 100, 94, 90, 84, 62, 50, 48, 46, 42, 38, 36, 36, 34, 32...
## $ n <dbl> 18, 11, 13, 14, 14, 10, 7, 6, 7, 6, 5, 5, 4, 6, 5, 5, 4, 5, ...
## $ n1 <dbl> 9, 7, 4, 5, 2, 3, 2, 3, 1, 2, 2, 1, 2, 1, 1, 0, 2, 2, 1, 1, ...
## $ n2 <dbl> 3, 3, 5, 3, 4, 1, 2, 2, 3, 1, 0, 1, 2, 0, 1, 3, 1, 0, 1, 1, ...
## $ n3 <dbl> 3, 1, 1, 1, 2, 1, 2, 0, 1, 1, 3, 3, 0, 2, 2, 1, 0, 1, 1, 1, ...
## $ n4 <dbl> 1, 0, 1, 0, 4, 4, 0, 0, 1, 2, 0, 0, 0, 3, 0, 0, 1, 0, 1, 0, ...
## $ n5 <dbl> 2, 0, 2, 5, 2, 1, 1, 1, 1, 0, 0, 0, 0, 0, 1, 1, 0, 2, 1, 2, ...
rankings <- rankings %>%
mutate(search_term = paste(title, artist)) %>%
mutate(search_term = str_to_lower(search_term)) %>%
mutate(search_term = str_remove(search_term, "ft.*$"))
spotify_search <- function(query){
spotifyr::search_spotify(query, type = 'track') %>%
filter(popularity == max(popularity)) %>%
pull(id)
}
spotify_search('Dear Mama')
## [1] "6tDxrq4FxEL2q15y37tXT9"
ranking_ids <- rankings %>%
mutate(id = map(search_term, possibly(spotify_search, NA_character_))) %>%
unnest(id)
ranking_ids %>%
na.omit() %>%
count(is.na(id), wt = n)
## # A tibble: 1 x 2
## `is.na(id)` n
## <lgl> <dbl>
## 1 FALSE 519
percent(mean(is.na(ranking_ids$id)))
## [1] "5%"
ranking_features <- ranking_ids %>%
mutate(id_group = row_number() %/% 80) %>%
select(id, id_group) %>%
nest(data = c('id')) %>%
mutate(audio_features = map(data, ~spotifyr::get_track_audio_features(.$id))) %>%
unnest(data, audio_features)
## Warning: unnest() has a new interface. See ?unnest for details.
## Try `df %>% unnest(c(data, audio_features))`, with `mutate()` if needed
ranking_df <- ranking_ids %>%
left_join(ranking_features) %>%
select(title, artist, points, year,
danceability:tempo) %>%
na.omit()
## Joining, by = "id"
library(corrr)
ranking_df %>%
select(year:tempo) %>%
correlate() %>%
rearrange() %>%
shave %>%
rplot()
##
## Correlation method: 'pearson'
## Missing treated using: 'pairwise.complete.obs'
## Registered S3 method overwritten by 'seriation':
## method from
## reorder.hclust gclus
## Don't know how to automatically pick scale for object of type noquote. Defaulting to continuous.
Lets use tidymodels
ranking_rec <- recipe(points ~ ., data = ranking_df) %>%
update_role(title, artist, new_role = "id") %>%
step_log(points) %>%
step_normalize(all_predictors()) %>%
step_pca(all_predictors())
ranking_prep <- prep(ranking_rec)
tidied_pca <- tidy(ranking_prep,3)
tidied_pca %>%
mutate(component = fct_inorder(component)) %>%
ggplot(aes(value, terms, fill = terms)) +
geom_col(show.legend = F) +
facet_wrap(~component) +
labs(y = NULL)
library(tidytext)
tidied_pca %>%
filter(component %in% c("PC1", "PC2", "PC3", "PC4", "PC5", "PC6")) %>%
group_by(component) %>%
top_n(6, abs(value)) %>%
ungroup() %>%
mutate(terms = reorder_within(terms, abs(value), component)) %>%
ggplot(aes(value, terms, fill = value>0), alpha = 0.2) +
geom_col() +
facet_wrap(~component, scales = "free_y") +
scale_y_reordered() +
labs(x = "Absolute Value of Contribution",
y = NULL,
fill = "Positive?")
juice(ranking_prep) %>%
ggplot(aes(PC1, PC2)) +
geom_point(alpha = 0.) +
geom_text(aes(label = title), check_overlap = T)
sdev <- ranking_prep$steps[[3]]$res$sdev
percent_variation <- sdev^2 / sum(sdev^2)
tibble(component = unique(tidied_pca$component),
percent_var = percent_variation) %>%
mutate(component = fct_reorder(component, -percent_var)) %>%
ggplot(aes(component, percent_var)) +
geom_col()
Lets do a linear regression now on PCA
pca_lm <- juice(ranking_prep) %>%
select(-artist, -title) %>%
lm(points ~ ., data = .)
summary(pca_lm)
##
## Call:
## lm(formula = points ~ ., data = .)
##
## Residuals:
## Min 1Q Median 3Q Max
## -1.52232 -0.58476 0.02525 0.39202 2.86961
##
## Coefficients:
## Estimate Std. Error t value Pr(>|t|)
## (Intercept) 1.93004 0.04837 39.905 <2e-16 ***
## PC1 0.07391 0.03282 2.252 0.025 *
## PC2 -0.04769 0.03708 -1.286 0.199
## PC3 -0.05605 0.04121 -1.360 0.175
## PC4 0.01458 0.04612 0.316 0.752
## PC5 0.01326 0.04678 0.283 0.777
## ---
## Signif. codes: 0 '***' 0.001 '**' 0.01 '*' 0.05 '.' 0.1 ' ' 1
##
## Residual standard error: 0.8419 on 297 degrees of freedom
## Multiple R-squared: 0.02864, Adjusted R-squared: 0.01229
## F-statistic: 1.751 on 5 and 297 DF, p-value: 0.1228